multi-asset
How to harness machine learning for investment strategies
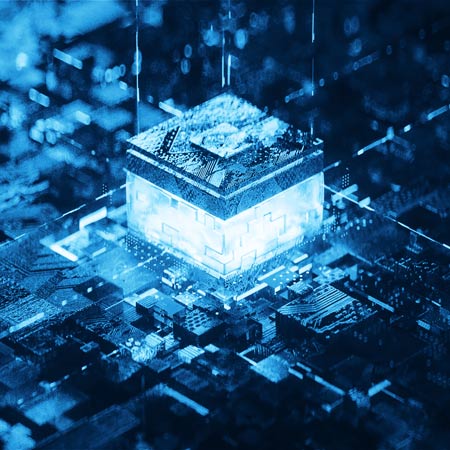


Need to know
|
---|
Successful partnership
Continuous investment research is a hallmark of the LOIM Multi Asset approach. It has spurred improvements in our risk-based strategies, recently enabling us to harness greater bond carry and to better integrate sustainability data in our investment process.
Since September 2020, LOIM has collaborated with data vendor Reuters/Refinitiv and French machine-learning start-up Ai for Alpha in a research partnership made possible by a grant from the European Institute of Innovation & Technology, which is an integral part of Horizon Europe.
Accomplishments and awards
The venture has produced several joint projects, with some notable outcomes including two forthcoming publications in AAMAS ALA 2021 workshop, Machine Learning Group (Springer Neural Computing and Applications Journal) and Mining Data for Financial Applications: 6th ECML PKDD Workshop, MIDAS 2021 (Springer).
- “Adaptive Supervised Learning for Volatility Targeting Models” by Eric Benhamou, David Saltiel, Serge Tabachnik, Corentin Bourdeix, François Chareyron, Beatrice Guez. It is the fifth-most downloaded paper on SSRN for the topic ‘machine learning’.
- “Adaptive Learning for Financial Markets Mixing Model-Based and Model-Free RL for Volatility Targeting” by Eric Benhamou, David Saltiel, Serge Tabachnik, Sui Kai Wong, François Chareyron.
We are pleased that the second publication won the Best Paper Award at the 2021 MIDAS/ECML-PKDD conference, and that in recent months both have ranked among the 10 most downloaded papers on SSRN for their subjects.
Investment application
Seeking investment applications from the two accepted papers, we have summarised the findings on machine learning allocation for volatility targeting portfolios in a white paper. It focuses on the implementation of two machine-learning-based methodologies to determine the optimal allocation between volatility-targeting models. The first is based on deep reinforcement learning (DRL) and the second on gradient boosting decision trees (GBDT).
Differentiated approach
We find these innovative methodologies to be intrinsically different due to their learning approaches: GBDT uses supervised learning, whereas DRL draws on unsupervised learning1. Both rely on adaptive machine learning methods to take the typical regime changes of volatility into account – but their approaches vary, marking a notable distinction between the two.
To read the white paper, please use the download button provided.
Learn more about our Multi Asset capabilities here.
Sources
1 In machine learning, a distinction can be made between supervised learning which uses labelled input and output data, and unsupervised learning which does not. Unsupervised learning works on its own to discover the inherent structure of unlabelled data.
important information.
This document is issued by Lombard Odier Asset Management (Europe) Limited, authorised and regulated by the Financial Conduct Authority (the “FCA”), and entered on the FCA register with registration number 515393.
Lombard Odier Investment Managers (“LOIM”) is a trade name.
This document is provided for information purposes only and does not constitute an offer or a recommendation to purchase or sell any security or service. It is not intended for distribution, publication, or use in any jurisdiction where such distribution, publication, or use would be unlawful. This material does not contain personalized recommendations or advice and is not intended to substitute any professional advice on investment in financial products. Before entering into any transaction, an investor should consider carefully the suitability of a transaction to his/her particular circumstances and, where necessary, obtain independent professional advice in respect of risks, as well as any legal, regulatory, credit, tax, and accounting consequences. This document is the property of LOIM and is addressed to its recipient exclusively for their personal use. It may not be reproduced (in whole or in part), transmitted, modified, or used for any other purpose without the prior written permission of LOIM. This material contains the opinions of LOIM, as at the date of issue.
Neither this document nor any copy thereof may be sent, taken into, or distributed in the United States of America, any of its territories or possessions or areas subject to its jurisdiction, or to or for the benefit of a United States Person. For this purpose, the term "United States Person" shall mean any citizen, national or resident of the United States of America, partnership organized or existing in any state, territory or possession of the United States of America, a corporation organized under the laws of the United States or of any state, territory or possession thereof, or any estate or trust that is subject to United States Federal income tax regardless of the source of its income.
Source of the figures: Unless otherwise stated, figures are prepared by LOIM.
Although certain information has been obtained from public sources believed to be reliable, without independent verification, we cannot guarantee its accuracy or the completeness of all information available from public sources.
Views and opinions expressed are for informational purposes only and do not constitute a recommendation by LOIM to buy, sell or hold any security. Views and opinions are current as of the date of this presentation and may be subject to change. They should not be construed as investment advice.
No part of this material may be (i) copied, photocopied or duplicated in any form, by any means, or (ii) distributed to any person that is not an employee, officer, director, or authorised agent of the recipient, without Lombard Odier Asset Management (Europe) Limited prior consent. In the United Kingdom, this material is a marketing material and has been approved by Lombard Odier Asset Management (Europe) Limited which is authorized and regulated by the FCA. ©2021 Lombard Odier IM. All rights reserved.